Seattle, WA, USA / June 18, 2024
Workshop on Media Forensics @ CVPR 2024
CVPR 2024
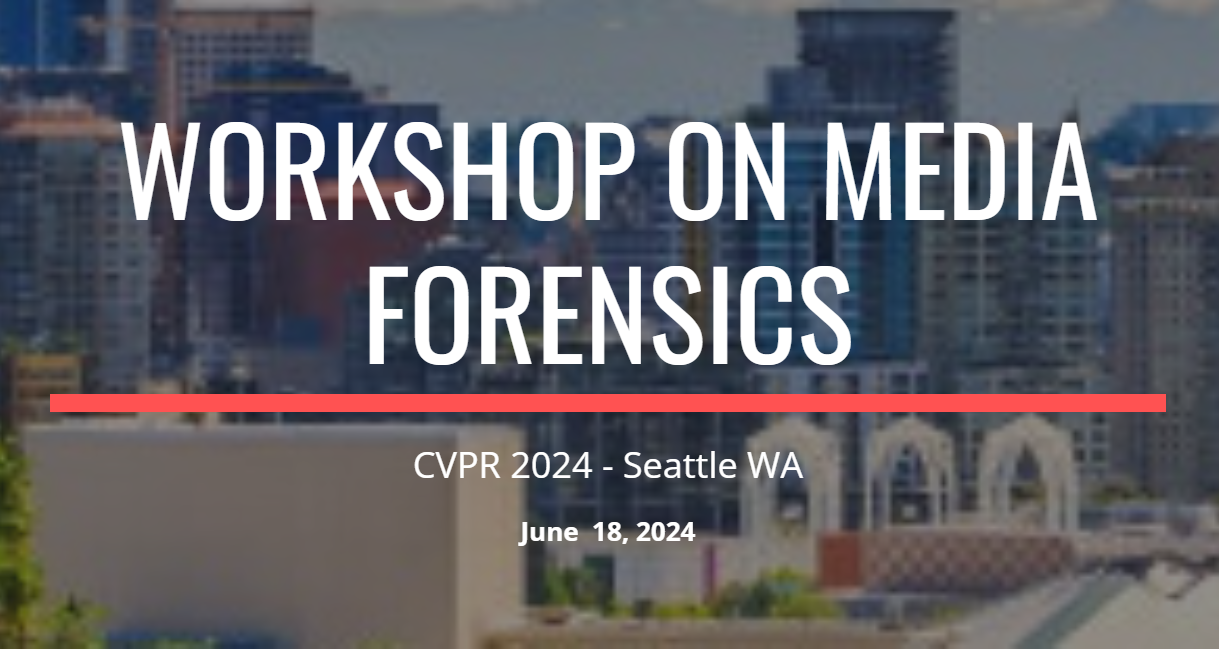
CVPR 2024
Workshop on Media Forensics @ CVPR 2024 will take place on June 18, 2024 in Seattle, WA, USA. With the emergence of more sophisticated ML and CV techniques, has multimedia forensics become a broad and prominent area of research. This workshop aims to bring together a heterogeneous group of specialists from academia and industry to discuss emerging threats, technologies, and mitigation strategies.
With contributions on synthetic speech detection and audio provenance analysis, Fraunhofer IDMT will present current research activities in the field of media forensics.
Milica Gerhardt, Luca Cuccovillo, Patrick Aichroth
This paper introduces a framework for Audio Provenance Analysis, addressing the complex challenge of analyzing heterogeneous sets of audio items without requiring any prior knowledge of their content. Our framework applies a novel approach that combines partial audio matching and phylogeny techniques. It constructs directed acyclic graphs to capture the origins and the evolution of content within near-duplicate audio clusters, identifying the least altered versions and tracing the reuse of content within these clusters. The approach is evaluated for two selected application scenarios, demonstrating that it can accurately determine the direction of content reuse and identify parent-child relationships, while also offering a dedicated dataset for benchmarking future research in this area.
Luca Cuccovillo, Milica Gerhardt, Patrick Aichroth
This paper introduces a novel multi-task transformer for detecting synthetic speech. The network encodes magnitude and phase of the input speech with a feature bottleneck, used to autoencode the input magnitude, to predict the trajectory of the first phonetic formants (F0, F1, F2), and to distinguish whether the input speech is synthetic or natural. The approach achieves state-of-the-art performance on the ASVspoof 2019 LA dataset with an AUC score of 0.932, while ensuring interpretability at the same time.